Want us to add your blog or article?
This site aggregates blogs and popular press articles about personality psychology. If you are an ARP member who writes a blog, or whose research has been featured in a recent popular press article, email us at personalitymetablog@gmail.com to have your work added to the meta-blog.-
Recent Posts
- Personality and Sheltering-in-place during the Pandemic – Sanjay Srivastava (Unique—Like Everybody Else)
- Who Supports Freedom of Speech? Tolerance vs. Prejudice – Sanjay Srivastava (Unique—Like Everybody Else)
- Who Uses Drugs and Why? – Sanjay Srivastava (Unique—Like Everybody Else)
- Why Religious People are Less Likely to Own Cats – Sanjay Srivastava (Unique—Like Everybody Else)
- Thoughts on “Ego Depletion” and Some Related Issues Concerning Replication – Sanjay Srivastava (funderstorms)
- The Real and Fake Faces of Personality’s ‘Big One’ – Sanjay Srivastava (Unique—Like Everybody Else)
Filter Posts by Blog
- citation needed (22)
- funderstorms (26)
- Person X Situation (4)
- pigee (35)
- Press coverage (3)
- Psych Your Mind (46)
- Secrets of Longevity (8)
- Sherman's Head (7)
- sometimes i'm wrong (66)
- The Desk Reject (10)
- The Hardest Science (55)
- The personality sentences (5)
- The SAPA Project (1)
- The Trait-State Continuum (34)
- Unique—Like Everybody Else (104)
Archives
Links to Contributing Blogs
- citation needed by Tal Yarkoni
- funderstorms by David Funder
- Person X Situation by Carol Tweten
- pigee by Brent Roberts
- Psych Your Mind coordinated by Michael Kraus
- Secrets of Longevity by Howard Friedman
- Sherman's Head by Ryne Sherman
- sometimes i'm wrong by Simine Vazire
- The Desk Reject Recent content on The Desk Reject
- The Hardest Science by Sanjay Srivastava
- The personality sentences by Jonathan Gerber
- The Trait-State Continuum by Brent Donnellan
- Unique—Like Everybody Else by Scott McGreal
Association for Research in Personality
Disclaimer
The views expressed in blog posts and other articles are those of the authors and do not necessarily reflect the views of the Association for Research in Personality.
Author Archives: Sanjay Srivastava
ASA releases consensus statement – Sanjay Srivastava (The Hardest Science)
Several months ago, the journal Basic and Applied Social Psychology published an editorial announcing a “ban” on p-values and confidence intervals, and treating Bayesian inferential methods with suspicion as well. The editorial generated quite a bit of buzz among scientists and statisticians alike.
In response the American Statistical Association released a letter expressing concern about the prospect of doing science without any inferential statistics at all. It announced that it would assemble a blue-ribbon panel of statisticians to issue recommendations.
That statement has now been completed, and I got my hands on an advance copy. Here it is:
We, the undersigned statisticians, represent the full range of statistical perspectives, Bayesian and frequentist alike. We have come to full agreement on the following points:
1. Regarding guiding principles, we all agree that statistical inference is an essential part of science and should not be dispensed with under any circumstances. Whenever possible you should put one of us on your grant to do it for you.
2. Continue reading
Comments Off on ASA releases consensus statement – Sanjay Srivastava (The Hardest Science)
Posted in The Hardest Science
Is there p-hacking in a new breastfeeding study? And is disclosure enough? – Sanjay Srivastava (The Hardest Science)
There is a new study out about the benefits of breastfeeding on eventual adult IQ, published in The Lancet Global Health. It’s getting lots of news coverage, for example in NPR, BBC, New York Times, and more.
A friend shared a link and asked what I thought of it. So I took a look at the article and came across this (emphasis added):
We based statistical comparisons between categories on tests of heterogeneity and linear trend, and we present the one with the lower p value. We used Stata 13·0 for the analyses. We did four sets of analyses to compare breastfeeding categories in terms of arithmetic means, geometric means, median income, and to exclude participants who were unemployed and therefore had no income.
Yikes. The description of the analyses is frankly a little telegraphic. But unless I’m misreading it, or they did some kind of statistical correction that they forgot to mention, it sounds like they had flexibility in the data analyses (I saw no mention of pre-registration in the analysis plan), they used that flexibility to test multiple comparisons, and they’re openly disclosing that they used p-values for model selection – which is a more technical way of saying they engaged in p-hacking. (They don’t say how they selected among the 4 sets of analyses with different kinds of means etc.; was that based on p-values too?)* Continue readingComments Off on Is there p-hacking in a new breastfeeding study? And is disclosure enough? – Sanjay Srivastava (The Hardest Science)
Posted in The Hardest Science
An open review of Many Labs 3: Much to learn – Sanjay Srivastava (The Hardest Science)
A pre-publication manuscript for the Many Labs 3 project has been released. The project, with 64 authors and supported by the Center for Open Science, ran replications of 10 previously-published effects on diverse topics. The research was conducted in 20 different university subject pools plus an Mturk comparison sample, with very high statistical power (over 3,000 subjects total). The project was pre-registered, and wherever possible the replicators worked with original authors or other experts to make the replications faithful to the originals. Big kudos to the project coordinating team and all the researchers who participated in this important work, as well as all the original authors who worked with the replicators.
A major goal was to examine whether time of semester moderates effect sizes, testing the common intuition among researchers that subjects are “worse” (less attentive) at the end of the term. But really, there is much more to it than that:
Not much replicated. The abstract says that 7 of 10 effects did not replicate. But dig a little deeper and the picture is more complicated. For starters, only 9 of those 10 effects were direct (or if you prefer, “close”) replications. The other was labeled a conceptual replication and deserves separate handling. More on that below; for now, let’s focus on the 9 direct replications.
Continue reading
Comments Off on An open review of Many Labs 3: Much to learn – Sanjay Srivastava (The Hardest Science)
Posted in The Hardest Science
Top 10 signs you are a statistics maven – Sanjay Srivastava (The Hardest Science)
I previously referenced Donald Sharpe’s idea of a statistics maven: people with one foot in a science field and one foot in statistics, who frequently act as a conduit for new quantitative innovations. Afterward I had an email exchange with someone who wanted to know how to become a maven, and I had to pass along the news that he probably already was. As a public service to others with similar concerns, I thought I should gather together the most probable symptoms (pending a comprehensive program of construct validation research, of course). Here at the top ten signs that you are a statistics maven:
10. You have installed R packages just to see what they do.
9. Your biggest regret from undergrad is a tossup between that person you never asked out and not taking more math.
8. You call the statistics you learned in grad school “frequentist statistics” and not just “statistics.”
7. People who are not quantitative psychologists call you a quantitative psychologist. Continue reading
Comments Off on Top 10 signs you are a statistics maven – Sanjay Srivastava (The Hardest Science)
Posted in The Hardest Science
Statistics as math, statistics as tools – Sanjay Srivastava (The Hardest Science)
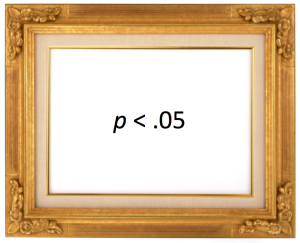
Comments Off on Statistics as math, statistics as tools – Sanjay Srivastava (The Hardest Science)
Posted in The Hardest Science
Popper on direct replication, tacit knowledge, and theory construction – Sanjay Srivastava (The Hardest Science)
I’ve quoted some of this before, but it was buried in a long post and it’s worth quoting at greater length and on its own. It succinctly lays out his views on several issues relevant to present-day discussions of replication in science. Specifically, Popper makes clear that (1) scientists should replicate their own experiments; (2) scientists should be able to instruct other experts how to reproduce their experiments and get the same results; and (3) establishing the reproducibility of experiments (“direct replication” in the parlance of our times) is a necessary precursor for all the other things you do to construct and test theories.
Kant was perhaps the first to realize that the objectivity of scientific statements is closely connected with the construction of theories — with the use of hypotheses and universal statements. Only when certain events recur in accordance with rules or regularities, as is the case with repeatable experiments, can our observations be tested — in principle — by anyone. We do not take even our own observations quite seriously, or accept them as scientific observations, until we have repeated and tested them. Only by such repetitions can we convince ourselves that we are not dealing with a mere isolated ‘coincidence’, but with events which, on account of their regularity and reproducibility, are in principle inter-subjectively testable.
Every experimental physicist knows those surprising and inexplicable apparent ‘effects’ which in his laboratory can perhaps even be reproduced for some time, but which finally disappear without trace. Of course, no physicist would say in such a case that he had made a scientific discovery (though he might try to rearrange his experiments so as to make the effect reproducible). Indeed the scientifically significant physical effect may be defined as that which can be regularly reproduced by anyone who carries out the appropriate experiment in the way prescribed. No serious physicist would offer for publication, as a scientific discovery, any such ‘occult effect,’ as I propose to call it — one for whose reproduction he could give no instructions. The ‘discovery’ would be only too soon rejected as chimerical, simply because attempts to test it would lead to negative results. (It follows that any controversy over the question whether events which are in principle unrepeatable and unique ever do occur cannot be decided by science: it would be a metaphysical controversy. Continue reading
Comments Off on Popper on direct replication, tacit knowledge, and theory construction – Sanjay Srivastava (The Hardest Science)
Posted in The Hardest Science
The selection-distortion effect: How selection changes correlations in surprising ways – Sanjay Srivastava (The Hardest Science)
A little while back I ran across an idea buried in an old paper of Robyn Dawes that really opened my eyes. It was one of those things that seemed really simple and straightforward once I saw it. But I’d never run across it before.[1] The idea is this: when a sample is selected on a combination of 2 (or more) variables, the relationship between those 2 variables is different after selection than it was before, and not just because of restriction of range. The correlation changes in ways that, if you don’t realize it’s happening, can be surprising and potentially misleading. It can flip the sign of a correlation, or turn a zero correlation into a substantial one. Let’s call it the selection-distortion effect.
First, some background: Dawes was the head of the psychology department at the University of Oregon back in the 1970s. Merging his administrative role with his interests in decision-making, he collected data about graduate admissions decisions and how well they predict future outcomes. He eventually wrote a couple of papers based on that work for Science and American Psychologist. The Science paper, titled “Graduate admission variables and future success,” was about why the variables used to select applicants to grad school do not correlate very highly with the admitted students’ later achievements. Dawes’s main point was to demonstrate why, when predictor variables are negatively correlated with each other, they can be perfectly reasonable predictors as a set even though each one taken on its own has a low predictive validity among selected students.
However, in order to get to his main point Dawes had to explain why the correlations would be negative in the first place. Continue reading
Comments Off on The selection-distortion effect: How selection changes correlations in surprising ways – Sanjay Srivastava (The Hardest Science)
Posted in The Hardest Science
Failed experiments do not always fail toward the null – Sanjay Srivastava (The Hardest Science)
There is a common argument among psychologists that null results are uninformative. Part of this is the logic of NHST – failure to reject the null is not the same as confirmation of the null. Which is an internally valid statement, but ignores the fact that studies with good power also have good precision to estimate effects.
However there is a second line of argument which is more procedural. The argument is that a null result can happen when an experimenter makes a mistake in either the design or execution of a study. I have heard this many times; this argument is central to an essay that Jason Mitchell recently posted arguing that null replications have no evidentiary value. (The essay said other things too, and has generated some discussion online; see e.g., Chris Said’s response.)
The problem with this argument is that experimental errors (in both design and execution) can produce all kinds of results, not just the null. Confounds, artifacts, failures of blinding procedures, demand characteristics, outliers and other violations of statistical assumptions, etc. can all produce non-null effects in data. When it comes to experimenter error, there is nothing special about the null. Continue reading
Comments Off on Failed experiments do not always fail toward the null – Sanjay Srivastava (The Hardest Science)
Posted in The Hardest Science
Tagged research methods
Some thoughts on replication and falsifiability: Is this a chance to do better? – Sanjay Srivastava (The Hardest Science)
Most psychologists would probably endorse falsification as an important part of science. But in practice we rarely do it right. As others have observed before me, we do it backwards. Instead of designing experiments to falsify the hypothesis we are testing, we look for statistical evidence against a “nil null” — the point prediction that the true effect is zero. Sometimes the nil null is interesting, sometimes it isn’t, but it’s almost never a prediction from the theory that we are actually hoping to draw conclusions about.
The more rigorous approach is to derive a quantitative prediction from a theory. Then you design an experiment where the prediction could fail if the theory is wrong. Statistically speaking, the null hypothesis should be the prediction from your theory (“when dropped, this object will accelerate toward the earth at 9.8 m/s^2″). Then if a “significant” result tells you that the data are inconsistent with the theory (“average measured acceleration was 8.6 m/s^2, which differs from 9.8 at p < .05″), you have to either set aside the theory itself or one of the supporting assumptions you made when you designed the experiment. You get some leeway to look to the supporting assumptions (“oops, 9. Continue reading
Comments Off on Some thoughts on replication and falsifiability: Is this a chance to do better? – Sanjay Srivastava (The Hardest Science)
Posted in The Hardest Science
Does the replication debate have a diversity problem? – Sanjay Srivastava (The Hardest Science)
Folks who do not have a lot of experiences with systems that don’t work well for them find it hard to imagine that a well intentioned system can have ill effects. Not work as advertised for everyone. That is my default because that is my experience.
– Bashir, Advancing How Science is Done
A couple of months ago, a tenured white male professor* from an elite research university wrote a blog post about the importance of replicating priming effects, in which he exhorted priming researchers to “Nut up or shut up.”
Just today, a tenured white male professor* from an elite research university said that a tenured scientist who challenged the interpretation and dissemination of a failed replication is a Rosa Parks, “a powerless woman who decided to risk everything.”
Well then.
The current discussion over replicability and (more broadly) improving scientific integrity and rigor is an absolutely important one. It is, at its core, a discussion about how scientists should do science. It therefore should include everybody who does science or has a stake in science.
Yet over the last year or so I have heard a number of remarks (largely in private) from scientists who are women, racial minorities, and members of other historically disempowered groups that they feel like the protagonists in this debate consist disproportionately of white men with tenure at elite institutions. Continue reading
Comments Off on Does the replication debate have a diversity problem? – Sanjay Srivastava (The Hardest Science)
Posted in The Hardest Science